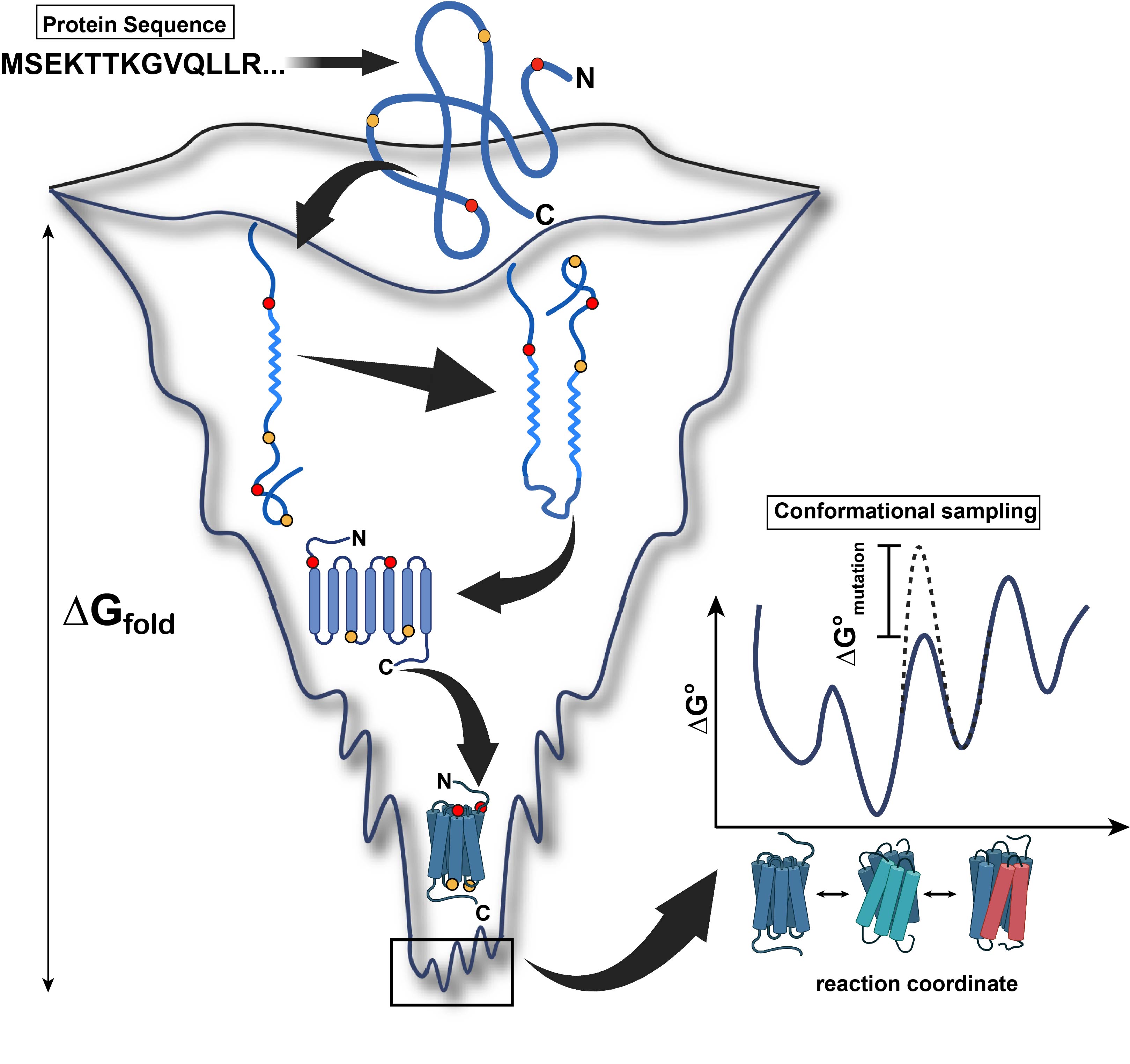
A key outstanding question in protein science has been the relationship between sequence and biological function. For decades since the central dogma became firmly rooted in biology, this “holy grail” type of question has been framed into a sequence/structure code, a structure/dynamics code and dynamic/function code. Up until recently (some would argue that even now) these codes have remained, by and large, recalcitrant to general principles. The recent transformational advances in artificial intelligence and machine learning technologies (hereafter collectively referred to as AI) is ushering in a new dawn in what we have often referred to as “mechanistic structural biology”, or structural biology in four dimensions. While falling short of capturing the sequence to function codes in the form of laws of physics, AI has proven efficient at implicitly decoding the manifestations of these codes embedded in evolutionary histories and known structures. The release of AlphaFold2 (AF2) and other AI structure prediction methods has galvanized protein scientists – witness the avalanche of publications and preprints and the constant stream of algorithms that harness the power of these approaches.
Dynamics make life possible. From birth, proteins undergo motions that enable them to sample the energy funnel en route to the native, folded ensemble of states. Lying at the bottom of the funnel, the free energy minimum consists of a rugged landscape of local minima separated by energy hills. Each of these minima represent a different conformation of the protein. Different type of dynamic modes, involving segments of secondary structures and domains, often at a relatively slower time scale, allow the protein to transition between these intermediates. Deciphering these dynamic modes, their thermodynamics and kinetics computationally is the next frontier in protein science and the goal around which the center was conceived.